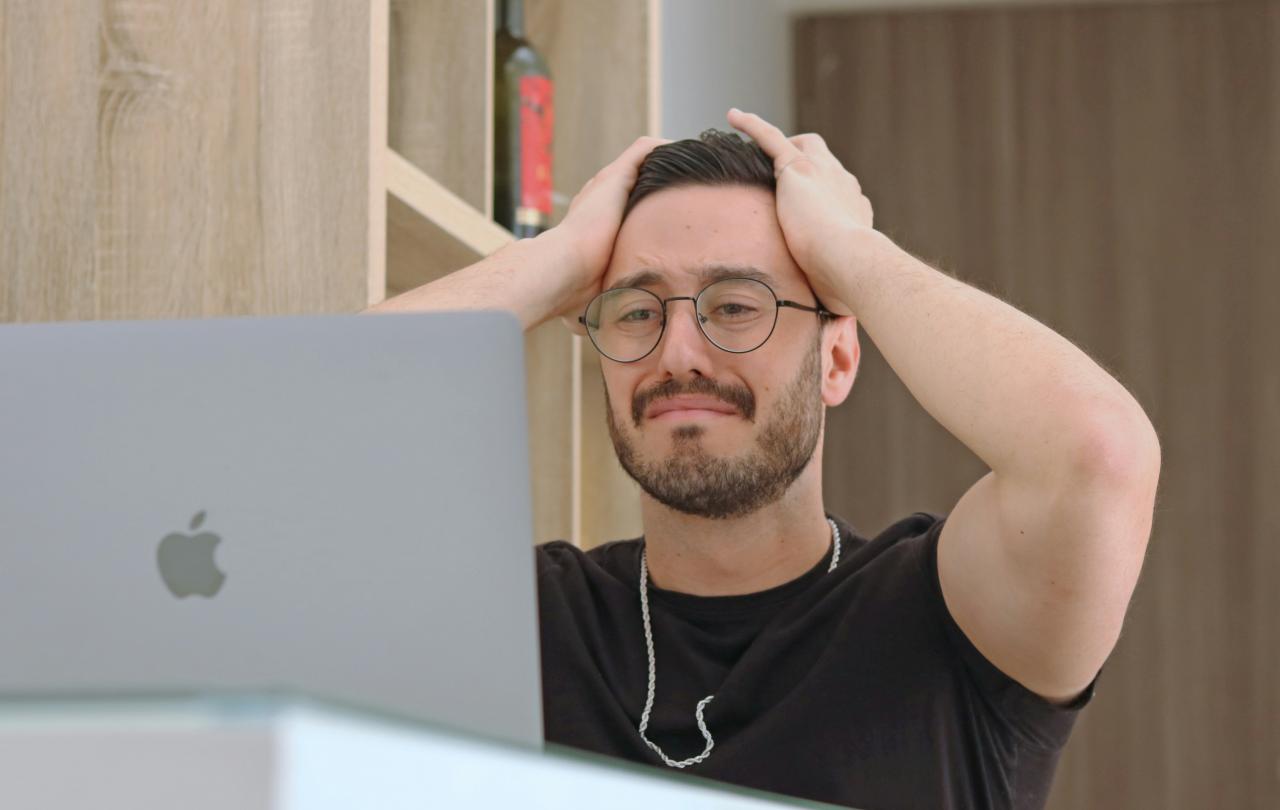
The distinction between technology and human beings has become blurry: AI seems to be able to listen, answer our questions, even respond to our feelings. It becomes increasingly easy to confuse machines with humans. In this situation, it is increasingly important to ask: What makes us human, in distinction from machines? There are many answers to this question, but for now I would like to focus on just one aspect of what I think is distinctively human: As human beings, we live and learn in time.
To be human means to be intrinsically temporal. We live in time and are oriented towards a future good. We are learning animals, and our learning is bound up with the taking of time. When we learn to know or to do something, we necessarily make mistakes, and we take practice. But keeping in view something we desire – a future good – we keep going.
Let’s take the example of language. We acquire language in community over time. Toddlers make all sorts of hilarious mistakes when they first try to talk, and it takes them a long time even to get single words right, let alone to try and form sentences. But they keep trying, and they eventually learn. The same goes with love: Knowing how to love our family or our neighbours near and far is not something we are good at instantly. It is not the sort of learning where you absorb a piece of information and then you ‘get’ it. No, we learn it over time, we imitate others, we practice and even when we have learned, in the abstract, what it is to be loving, we keep getting it wrong.
This, too, is part of what it means to be human: to make mistakes. Not the sort of mistakes machines make, when they classify some information wrongly, for instance, but the very human mistake of falling short of your own ideal. Of striving towards something you desire – happiness, in the broadest of terms – and yet falling short, in your actions, of that very goal. But there’s another very human thing right here: Human beings can also change. They – we – can have a change of heart, be transformed, and at some point in time, actually start to do the right thing – even against all the odds. Statistics of past behaviours, do not always correctly predict future outcomes. Part of being human means that we can be transformed.
Transformation sometimes comes suddenly, when an overwhelming, awe-inspiring experience changes somebody’s life as by a bolt of lightning. Much more commonly, though, such transformation takes time. Through taking up small practices, we can form new habits, gradually acquire virtue, and do the right thing more often than not. This is so human: We are anything but perfect. As Christians would say: We have a tendency to entangle ourselves in the mess of sin and guilt. But we also bear the image of the Holy One who made us, and by the grace and favour of that One, we are not forever stuck in the mess. We are redeemed: are given the strength to keep trying, despite the mistakes we make, and given the grace to acquire virtue and become better people over time. All of this to say that being human means to live in time, and to learn in time.
So, this is a real difference between human beings and machines: Human beings can, and do strive toward a future good.
Now compare this to the most complex of machines. We say that AI is able to “learn”. But what does it mean to learn, for AI? Machine learning is usually categorized into supervised learning, unsupervised and self-supervised learning. Supervised learning means that a model is trained for a specific task based on correctly labelled data. For instance, if a model is to predict whether a mammogram image contains a cancerous tumour, it is given many example images which are correctly classed as ‘contains cancer’ or ‘does not contain cancer’. That way, it is “taught” to recognise cancer in unlabelled mammograms. Unsupervised learning is different. Here, the system looks for patterns in the dataset it is given. It clusters and groups data without relying on predefined labels. Self-supervised learning uses both methods: Here, the system uses parts of the data itself as a kind of label – such as, for instance, predicting the upper half of an image from its lower half, or the next word in a given text. This is the predominant paradigm for how contemporary large-scale AI models “learn”.
In each case, AI’s learning is necessarily based on data sets. Learning happens with reference to pre-given data, and in that sense with reference to the past. It may look like such models can consider the future, and have future goals, but only insofar as they have picked up patterns in past data, which they use to predict future patterns – as if the future was nothing but a repetition of the past.
So this is a real difference between human beings and machines: Human beings can, and do strive toward a future good. Machines, by contrast, are always oriented towards the past of the data that was fed to them. Human beings are intrinsically temporal beings, whereas machines are defined by temporality only in a very limited sense: it takes time to upload data, and for the data to be processed, for instance. Time, for machines, is nothing but an extension of the past, whereas for human beings, it is an invitation to and the possibility for being transformed for the sake of a future good. We, human beings, are intrinsically temporal, living in time towards a future good – which machines do not.
In the face of new technologies we need a sharpened sense for the strange and awe-inspiring species that is the human race, and cultivate a new sense of wonder about humanity itself.