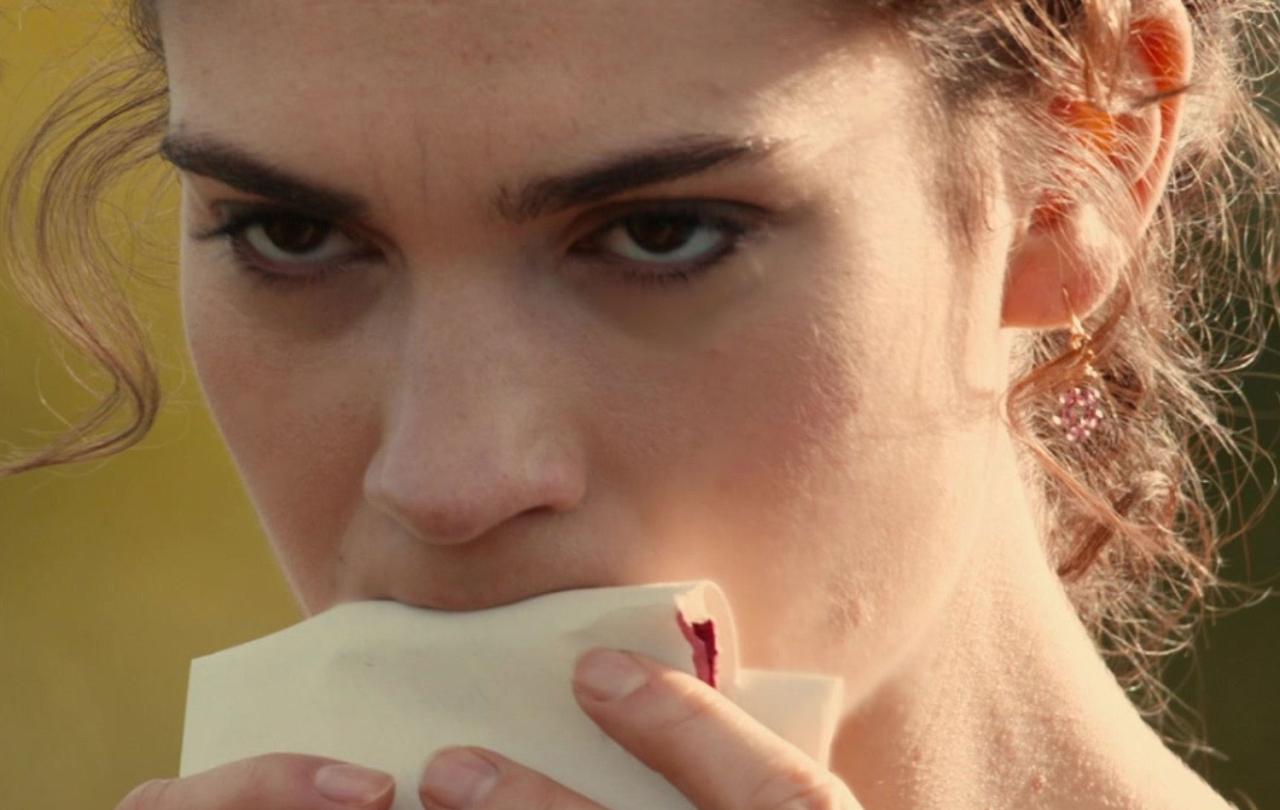
Writing from his new book, A Guidebook to Monsters, Ryan Stark delves into humanity’s fascination for all things monsterous. In the second of a two-part series, he asks what and where zombies remind us of, and why they caught the eyes of C.S. Lewis and Salvador Dali
On how Frankenstein’s monster came to life nobody knows for sure, but he is more urbane than zombies tend to be. Nor do Jewish golems and Frosty the Snowman count as zombiecore. The latter sings too much, and both are wrongly formulated. Frosty comes from snow, obviously, and the golems—from mere loam, not what the Renaissance playwrights call “gilded loam,” that is, already pre-assembled bodies, which is a zombie requirement. Tolkien’s orcs function likewise as golem-esque monsters, cast from miry clay and then enlivened by the grim magic of Mordor. We do not, for instance, discover scenes with orc children.
And neither is Pinocchio a zombie, nor Pris from Blade Runner, but dolls, automatons, and C3POs border upon the land of zombies insofar as they all carry a non-human tint. Zombies, however, carry something else as well, a history of personhood, and so in their present form appear as macabre parodies of the human condition writ large. They are gruesome undead doppelgangers, reminding us of who we are not and perhaps—too—of where we are not. Hell is a place prepared for the Devil and his angels, Christ tells us in the book of Matthew. And maybe, subsequently, for zombies.
Kolchak, in an episode of Kolchak: The Night Stalker aptly titled “The Zombie,” correctly discerns the grim scenario at hand: “He, sir, is from Hell itself!”
C.S. Lewis pursues a similar line of thinking in The Problem of Pain: “You will remember that in the parable, the saved go to a place prepared for them, while the damned go to a place never made for men at all. To enter Heaven is to become more human than you ever succeeded in being on earth; to enter Hell is to be banished from humanity. What is cast (or casts itself) into Hell is not a man: it is ‘remains.’” Lewis makes an intriguing point, which has as its crescendo the now-famous line about the doors of Hell: “I willingly believe that the damned are, in one sense, successful, rebels to the end; that the doors of Hell are locked on the inside by zombies.” I added that last part about zombies.
I make this point—in part—to correct those in the cognoscenti who dismiss zombies as a subject too lowbrow for serious consideration.
Not everyone believes in Hell, of course, yet most concede that some people behave worse than others, which also helps our cause. Indeed, part of zombiecore’s wisdom is to show that bad people often produce more horror than the zombies themselves. Such is the character of Legendre Murder, a case in point from the film White Zombie. Not fortunate in name, Mr. Murder runs a dark satanic mill populated by hordes of zombie workers, which is the film’s heavy-handed critique of sociopathic industrialization. The truth to be gleaned, here, is that zombies did not invent the multinational corporation; rather, they fell prey to it.
We might think, too, of Herman Melville’s dehumanized characters from Bartleby the Scrivener: Nippers, Turkey, Ginger Nut, and the other functionaries whose nicknames themselves indicate the functions. From an economic standpoint, their value becomes a matter of utility, not essence, which is Melville’s reproach of the despairingly corporate drive to objectify personhood—of which zombies are an example beyond the pale. They might as well be fleshy mannequins, in fact, and as such provide the perfect foil for the human being properly conceived.
Here, then, is why we do not blame zombies for eating brains, nor do we hold them accountable for wearing white pants after Labor Day, as some inevitably do. They cannot help it—in ethics and in fashion. Perhaps especially in fashion. The best we can hope for in the realm of zombie couture is Solomon Grundy, the quasi-zombie supervillain who holds up his frayed pants with a frayed rope, a fashion victory to be sure, however small it might be, though “zombie fashion” is a misnomer in the final analysis. They wear clothes, but not for the same reasons we do.
The point holds true for Salvador Dali’s zombies as well, most of whom find themselves in nice dresses. I make this point—in part—to correct those in the cognoscenti who dismiss zombies as a subject too lowbrow for serious consideration. Not so. Exhibit A: the avant-garde Dali, darling of the highbrow, or at least still of the middlebrow, now that his paintings appear on t-shirts and coffee mugs. Burning giraffe. Mirage. Woman with Head of Roses. All zombies, too ramshackle and emaciated to live, never mind the missing head on the last one, and yet there they are posed for the leering eye, not unlike those heroin-chic supermodels from Vogue magazine in the late 1990s. Necrophilia never looked so stylish.
The zombie’s gloomy predicament bears a striking resemblance to that of the Danaids in the classical underworld, those sisters condemned to fill a sieve with water for all eternity...
But never let it be said that zombies are lazy. They are tired, to be sure. Their ragged countenances tell us this, but they are not indolent. Zombies live purpose-driven undead lives. They want to eat brains, or any human flesh, depending on the mythos, and their calendars are organized accordingly. No naps. No swimming lessons. Just brains.
But we quickly discern that no amount of flesh will satisfy. There is always one more hapless minimart clerk to ambush, one more sorority girl in bunny slippers to chase down the corridor. In this way, the zombie’s gloomy predicament bears a striking resemblance to that of the Danaids in the classical underworld, those sisters condemned to fill a sieve with water for all eternity, an emblem of the perverse appetite unchecked, which has at its core the irony of insatiable hunger. And as the pleasure becomes less and less, the craving becomes more and more. The law of diminishing returns. So, it is with all vices. The love of money demands more money, and the love of brains, more brains.
And so, in conclusion, a prayer. God bless the obsessive-compulsive internet shoppers, the warehouse workers on unnecessarily tight schedules, and the machine-like managers of the big data algorithms. God bless the students who sedate themselves in order to survive their own educations, taking standardized test after standardized test. And God bless the Emily Griersons of the world, who keep their petrified-boyfriend corpses near them in the bedroom, an emblem of what happens when one tries too mightily to hold on to the past. And God help us, too, when we see in our own reflections a zombie-like affectation, the abyss who stares back at us and falsely claims that we are not the righteousness of God, as Paul says we are in 2 Corinthians. And, finally, Godspeed to Gussie Fink-Nottle from the P.G. Wodehouse sagas: “Many an experienced undertaker would have been deceived by his appearance, and started embalming on sight.”
From A Guidebook to Monsters, Ryan J. Stark. Used by permission of Wipf and Stock Publishers.